일 | 월 | 화 | 수 | 목 | 금 | 토 |
---|---|---|---|---|---|---|
1 | 2 | |||||
3 | 4 | 5 | 6 | 7 | 8 | 9 |
10 | 11 | 12 | 13 | 14 | 15 | 16 |
17 | 18 | 19 | 20 | 21 | 22 | 23 |
24 | 25 | 26 | 27 | 28 | 29 | 30 |
- pytorch
- Meta Learning
- Python
- math
- PRML
- nlp
- REACT
- Front
- GAN
- Depth estimation
- dl
- 3d
- 알고리즘
- 머신러닝
- 딥러닝
- SSL
- FineGrained
- classification
- cs
- nerf
- 자료구조
- algorithm
- FGVC
- clean code
- computervision
- ML
- web
- Torch
- CV
- Vision
- Today
- Total
목록CV (110)
KalelPark's LAB
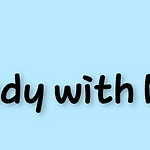
아래의 방법을 활용하면, 인용을 더 많이 할 수 있습니다 :) @STRING{TPAMI= "IEEE Transactions on Pattern Analysis and Machine Intelligence"} @STRING{IJCV= "International Journal of Computer Vision"} @STRING{TIP= "IEEE Transactions on Image Processing"} @STRING{TIT= "IEEE Transactions on Information Theory"} @STRING{CVIU= "Computer Vision and Image Understanding"} @STRING{PR= "Pattern Recognition"} @STRING{JMLR= "Journal..
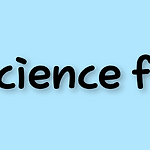
Load Library import torch import timm import numpy as np from einops import repeat, rearrange from einops.layers.torch import Rearrange from timm.models.layers import trunc_normal_ from timm.models.vision_transformer import Block Patch shuffle을 하기 위한 Class 및 function 구축 * forward_indexes : (16, 2) * backward_indexes : (16, 2) 이후, take_indexes를 거칠 때, gather는 각 차원의 vector를 0을 axis로 사용하므로, depth, c..
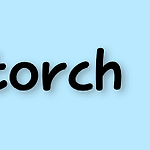
매번 까먹어서, 다시 다듬어보고자 한다. torch.gather란? 공식문서에 따르면 차원에 해 정해진 축에 따라 값을 모읍니다. import torch t = torch.tensor([[1, 2], [3, 4]]) torch.gather(t, 1, torch.tensor([[0, 0], [1, 0]])) 즉, 차원에 따라 값을 재배치한다고 이해하면 됩니다. * 코드로 한번 더 이해해보도록 하겠습니다. out[i][j][k] = input[index[i][j][k]][j][k] out[i][j][k] = input[i][index[j][k]][k] out[i][j][k] = input[i][j][index[k]] // 위의 값처럼 indexing이 처리되는 것을 알 수 있습니다. Example> imp..
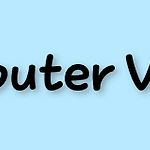
Abstract 기존 방법론들은 training distribution에 적합한 성능을 달성하였지만, 학습된 네트워크는 과적합되기 쉽고, 적대적 공격에 취약합니다. 이러한 문제를 해결하기 위해, Mixed 기반 Augmentation이 대두가 되지만, 이것은 이전에 인지하지 못한 객체를 인지하는데 초점을 두어, 모델에 오히려 오해의 소지를 제안할 수 있습니다. 이러한 문제를 해결하고자, PuzzleMix를 본 논문에서 제안합니다. 그러므로, 주변 환경정보를 활용하는 학습하는 방법론인 PuzzleMix를 제안합니다. 이는 optimal mixing mask와 saliency discounted optimal transport obejective 사이를 최적화하는 방식으로 문제를 해결하고자 합니다. Intr..
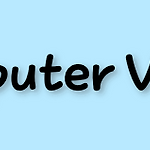
GitHub를 참고하시면, CODE 및 다양한 논문 리뷰가 있습니다! 하단 링크를 참고하시기 바랍니다. (+ Star 및 Follow는 사랑입니다..!) https://github.com/kalelpark/Awesome-ComputerVision GitHub - kalelpark/Awesome-ComputerVision: Awesome-ComputerVision Awesome-ComputerVision. Contribute to kalelpark/Awesome-ComputerVision development by creating an account on GitHub. github.com Abstract 이미지로부터의 Self Supervised Learning의 목표는 대규모 데이터에 대한 annotat..
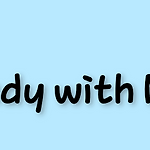
Comparison, Generator Comparison을 사용하면, 타입을 간결하게 이터레이션하면서 원소로부터 파생되는 데이터 구조를 생성할 수 있습니다. Comparison은 Generator로 확장하는 것이 가능합니다. Generator는 성능을 향상시키고, 메모리 사용량을 줄이고, 코드의 가독성을 향상시킬 수 있습니다. list comparision을 사용하는 경우, map과 filter를 사용하는 대신에, list에서 바로 생성하는 것이 좋습니다. alt = map(lambda x : x ** 2, a) # don't use even_square = [x ** 2 for x in a if x % 2 == 0] # using list comparison print(even_sqaure) Sequ..
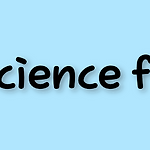
Logger - 서버에서 프로그램을 돌릴 때, 알아보기 쉽게 많은 것을 저장해두는 것이 좋습니다. print를 단순하기 사용하기 보다는, logger를 활용해서 저장해두는 것이 좋습니다. - 만약 loggging 시, text 파일을 이어쓰고자 한다면, mode를 "w"가 아닌 "a"를 사용하면 됩니다. wandb와 같이 어떻게 보면 유용하게 활용할 수 있습니다. 저는 이전에 tqdm을 사용하는데, 이제는 logger를 사용하려고 합니다. import sys class Logger(object): def __init__(self, local_rank = 0, no_save = False): self.terminal = sys.stdout self.file = None self.local_rank = lo..
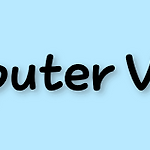
Abstract 본 논문에서는 Self-supervised Learning으로 확장가능한 masked autoencoder를 소개합니다. input 이미지를 무작위로 patch로 분할 한 후, 몇 개는 정보를 잃게 한후, 다시 생성하도록 학습하는 방식입니다. 주된 기여로는 2가지가 존재합니다. 1. Using the Encoder Decoder structure, and reconsturcture msising patches in decoder. (Encode, Decoder 구조를 사용합니다.) 2. we mask high propotion for self-supervised learning task. (상당히 많은 부분 75%를 missing patches로 활용합니다.) Introduction GP..