일 | 월 | 화 | 수 | 목 | 금 | 토 |
---|---|---|---|---|---|---|
1 | 2 | 3 | 4 | |||
5 | 6 | 7 | 8 | 9 | 10 | 11 |
12 | 13 | 14 | 15 | 16 | 17 | 18 |
19 | 20 | 21 | 22 | 23 | 24 | 25 |
26 | 27 | 28 | 29 | 30 | 31 |
- Vision
- Python
- GAN
- CV
- 머신러닝
- nerf
- REACT
- cs
- PRML
- web
- ML
- clean code
- 3d
- Front
- algorithm
- FGVC
- FineGrained
- math
- Depth estimation
- Torch
- 알고리즘
- dl
- 딥러닝
- nlp
- classification
- computervision
- Meta Learning
- pytorch
- SSL
- 자료구조
- Today
- Total
KalelPark's LAB
[Math] chapter 3.2 [Structure-from-Motion: Two-frame Structure-from-Motion] 본문
[Math] chapter 3.2 [Structure-from-Motion: Two-frame Structure-from-Motion]
kalelpark 2023. 4. 7. 20:01Epipolar Geometry
동일한 사물 또는 장면에 대한 영상을 서로 다른 두 지점에서 획득하였을 때, 영상 A와 영상 B의 매칭쌍들 사이의 기하학적 관계를 다루는 것을 의미합니다.
R 과 T는 각각 회전 행렬(Rotation Matrix), 평행 이동 벡터(translation vector)를 나타내는 변수입니다.
x와 2개의 카메라의 원점을 이은 평면은 epipolar plane이라고 하며, 카메라 원점과 x를 이은 선을 epipole이라고 합니다.
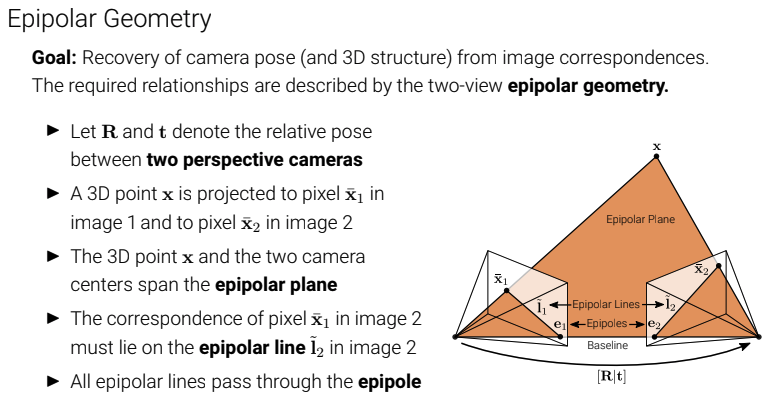
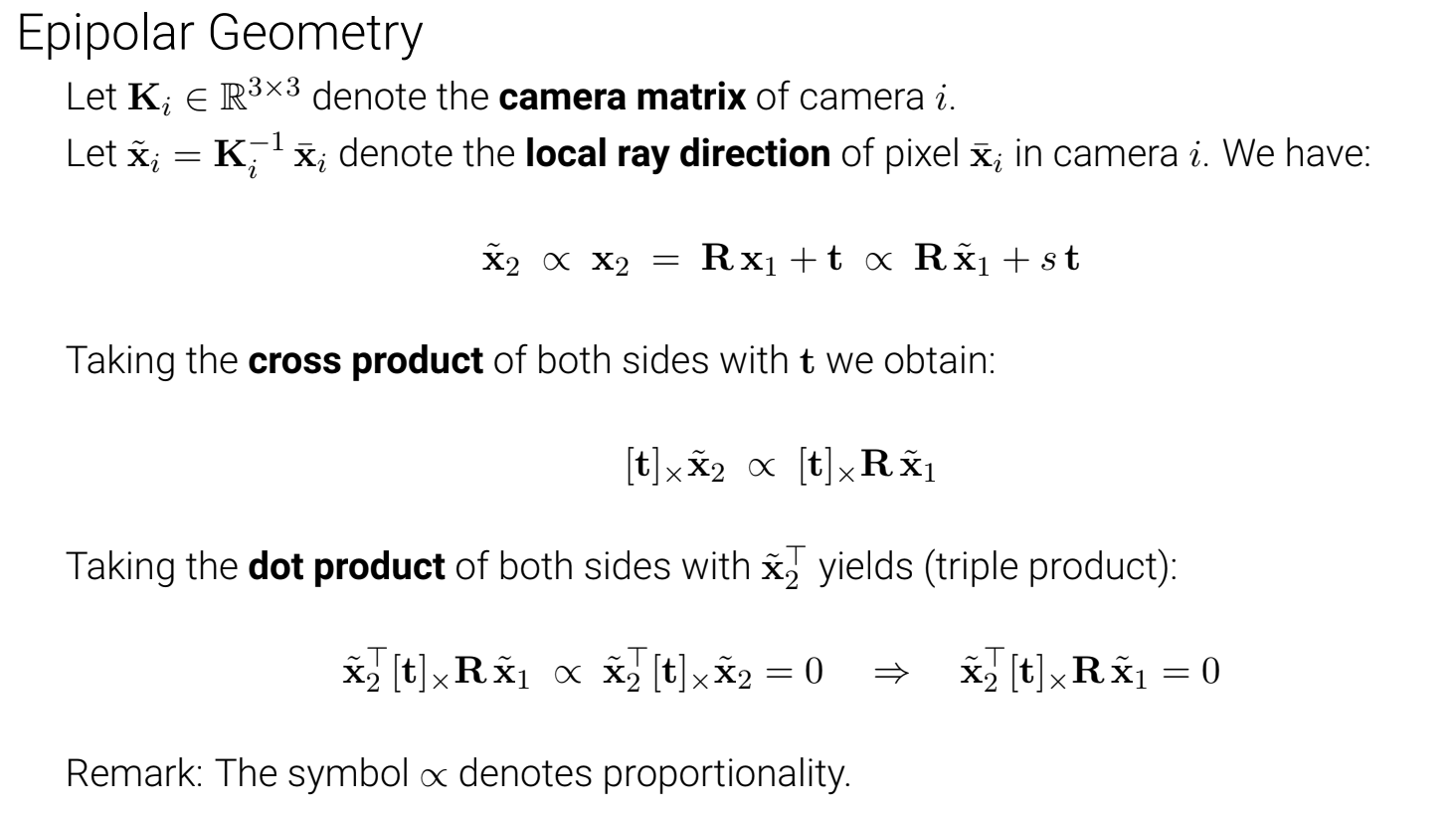
x2 x Rx = 0 이라면, x*Ex라고 할 수 있고, 그렇다면 이 E를 essential matrix라고 하고, epipolar contsraint라고 할 수 있습니다. Epipolar constraint는 Epipolar line 위에 존재하는 포인트가 다른 카메라의 이미지 평면 상에 위치할 때 해당 포인트가 취할 수 있는 가능한 위치에 대한 제약 조건을 나타냅니다.
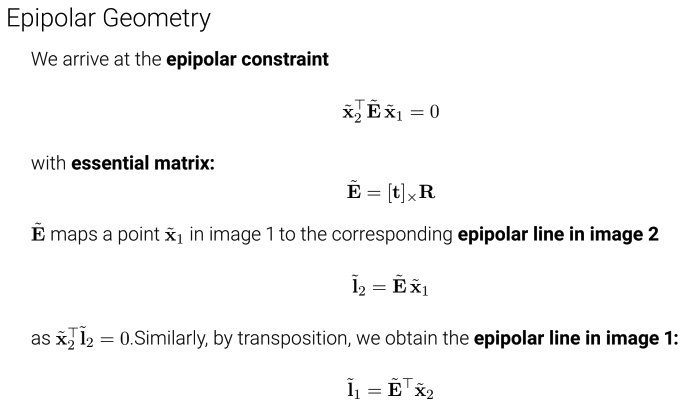
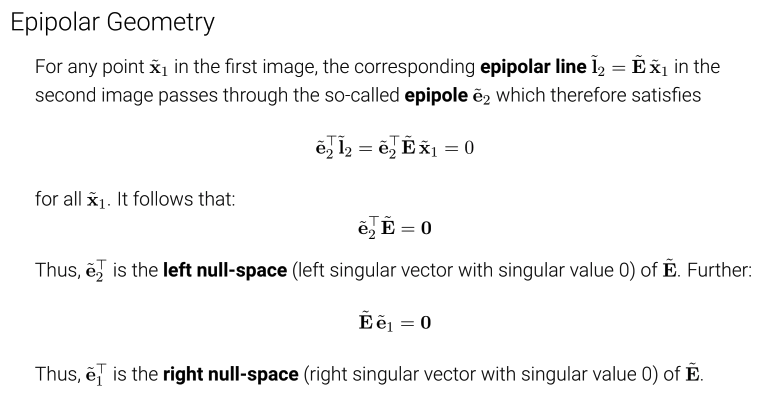
Estimating the Epipolar Geometry
우리는 E의 9개 요소에서 N개의 homogenous equation을 생성하는 N개의 이미지로부터 essential matrix를 생성하는 것이 가능합니다. E homogeneous를 사용함으로써, 우리는 singular value decomposition을 활용하여, scale을 constrain 할 수 있습니다.
E를 활용하여 T를 구할 수 있습니다. 여기서 E란 essential matrix라고 하며, 카메라 2대 위치와 대응되는 이미지 포인트들 사이의 관계를 나타내는 행렬을 의미합니다.
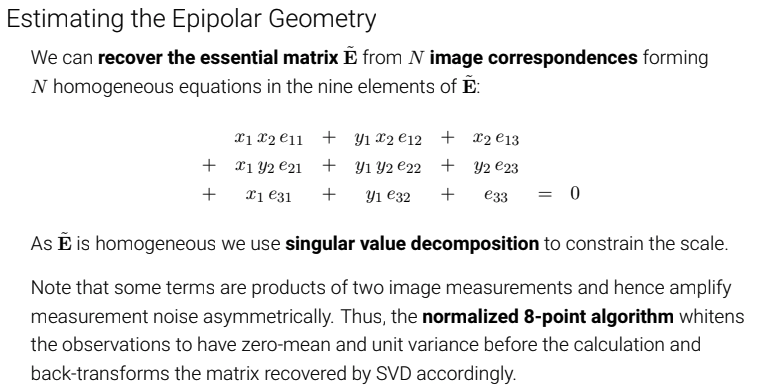
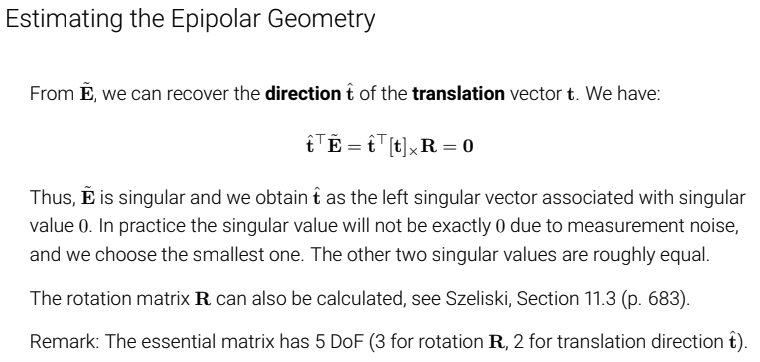
Estimating the Epipolar Geometry with unknown Intrinsics
만약 Camera Calibration K를 알 수 없다면, 우리는 x = kx를 pixel coordinates x로만으로 알 수 없습니다. 그러므로 Essential Matrix는 fundamental matrix라 불리는, F = k^(T)ek^(-1)가 됩니다. 즉, 카메라 파라미터(intrinsic parameters)가 없을 경우 우리는 대응 관계를 나타내는 fundamental matrix를 사용하여, 값을 구합니다.
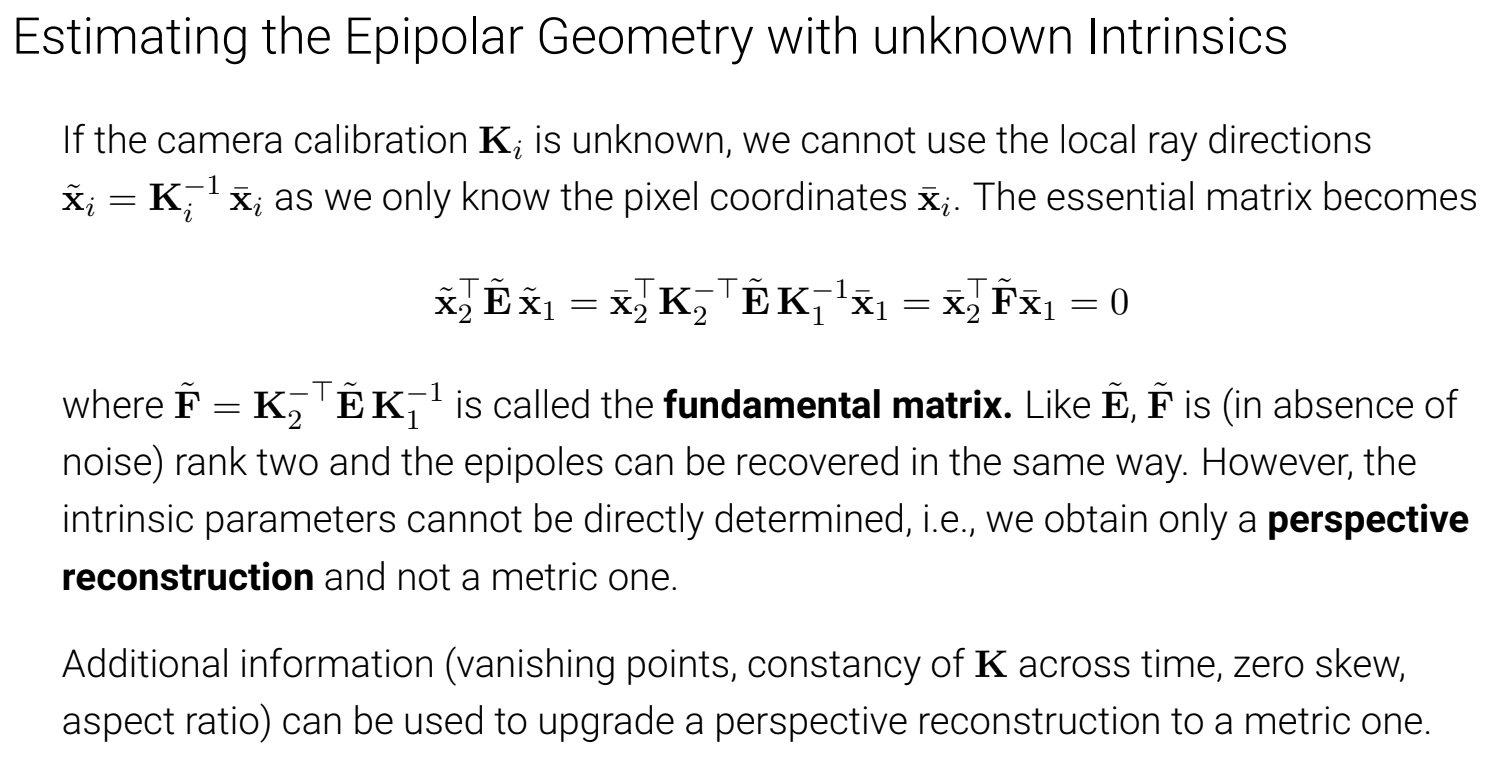
Triangulation
Camera내 intrinsics 과 extrinsics를 알 때, 어떻게 3D gemetry를 추정할 수 있을까? 2D image로 관찰한 x1과 x2를 고려하였을 때, two ray는 동일한 점에 위치하지 않습니다. 좌측 수식을 3D workd point를 카메라 위치에 투영하여 표현한 식입니다. 하지만, least square problem으로 인하여, 한 점이 나오지 않을 수 있습니다.
그러므로, 오차를 최소화하는 함수를 정의해서 더 정확한 값으로 갱신해야 합니다.
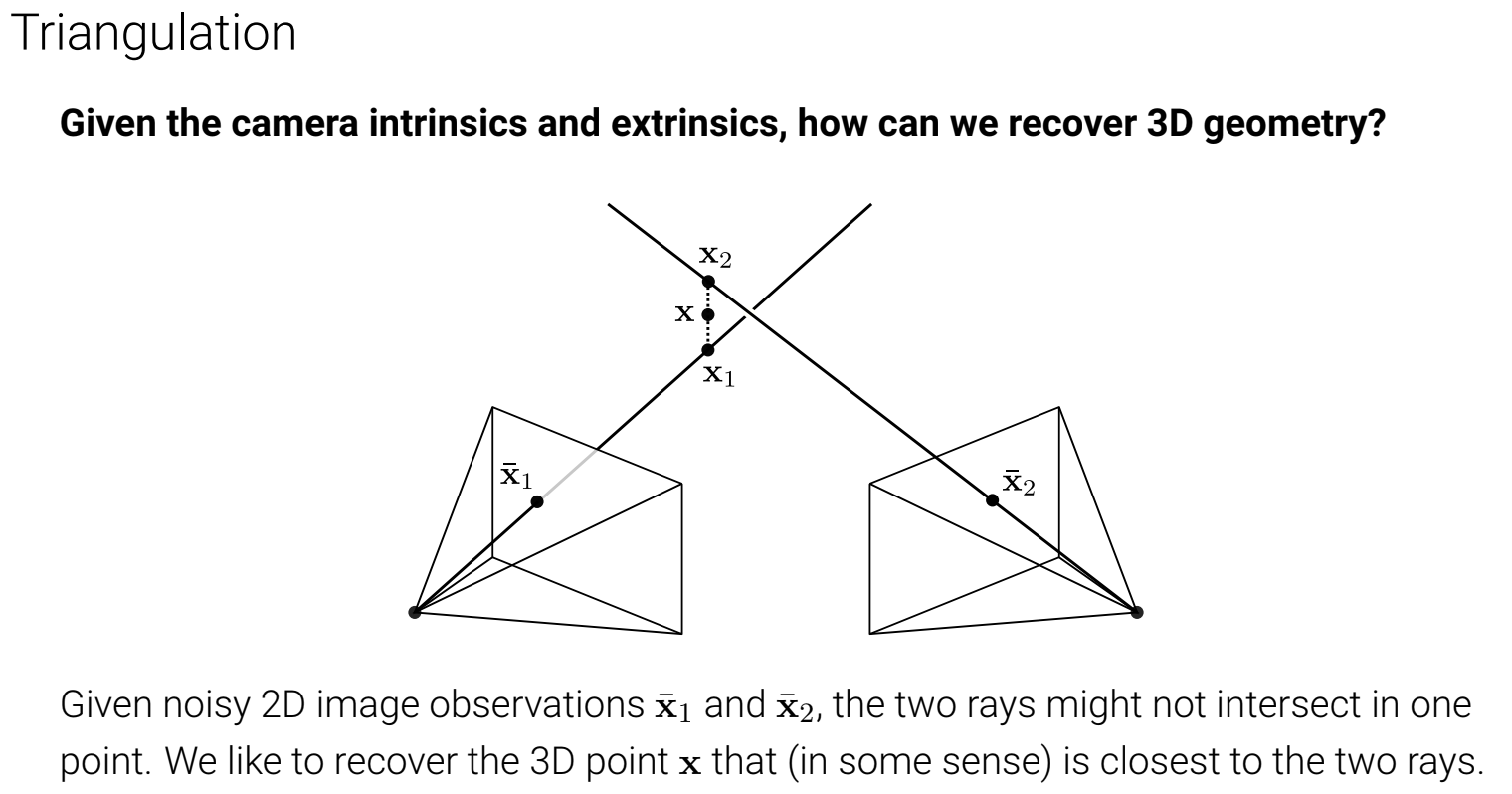
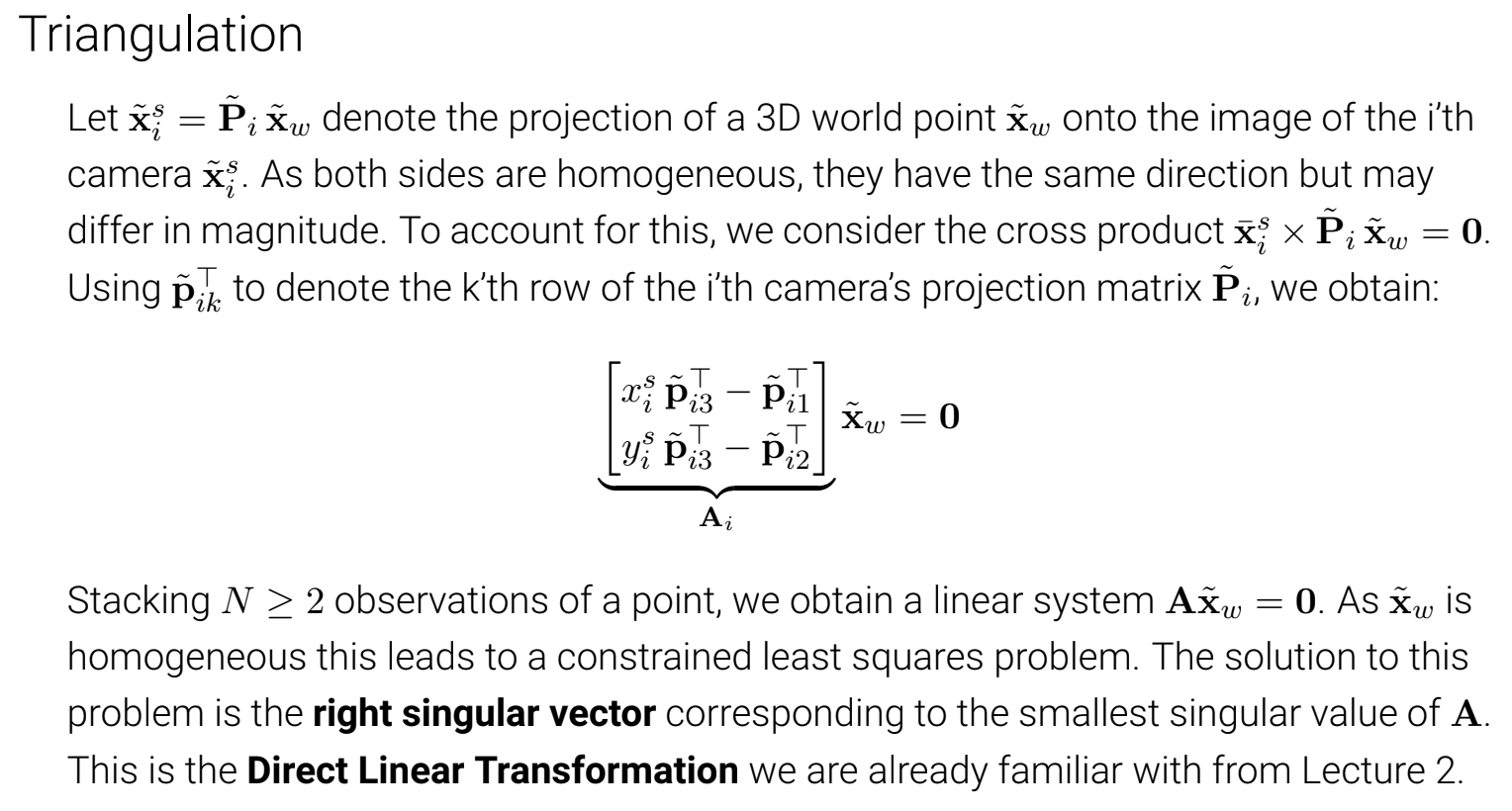
Triangulation
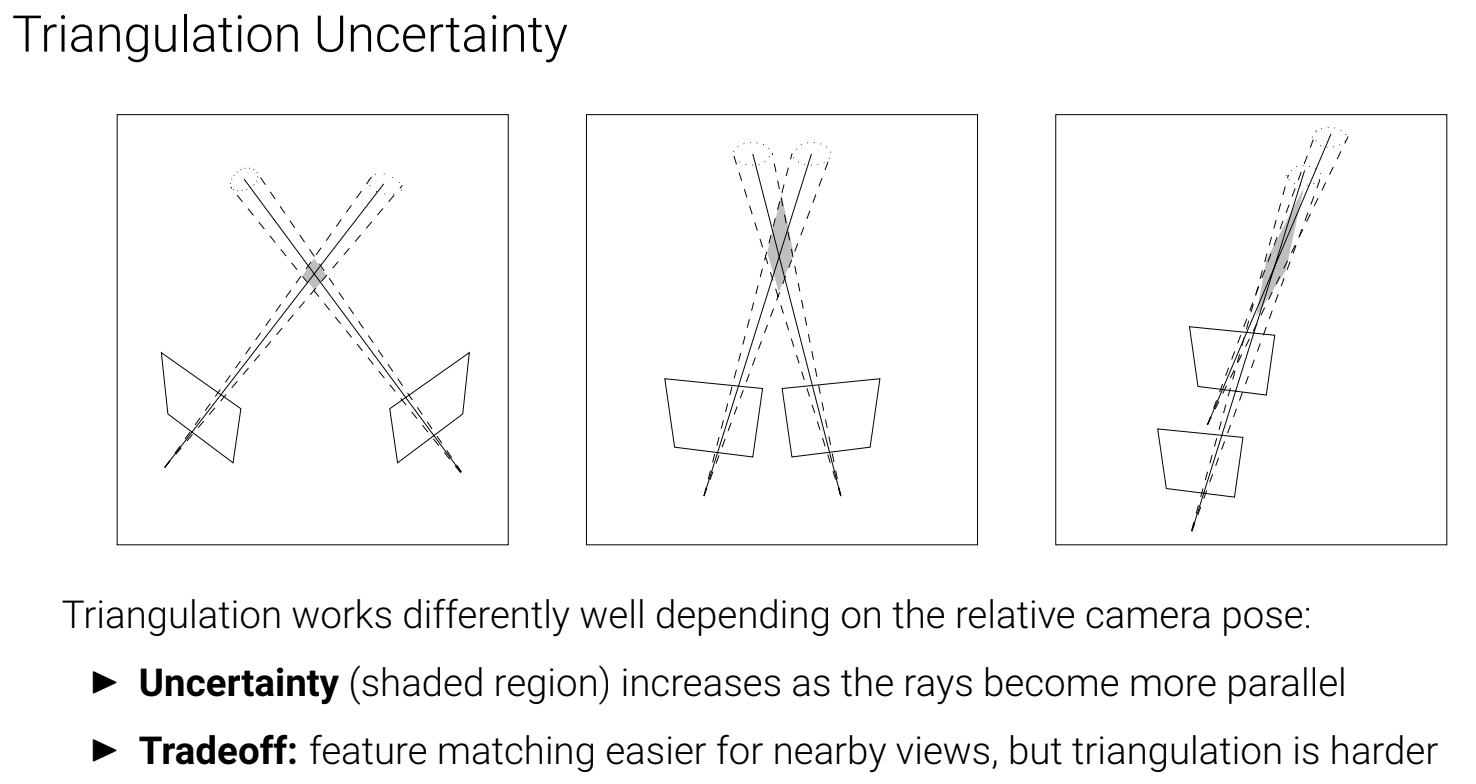
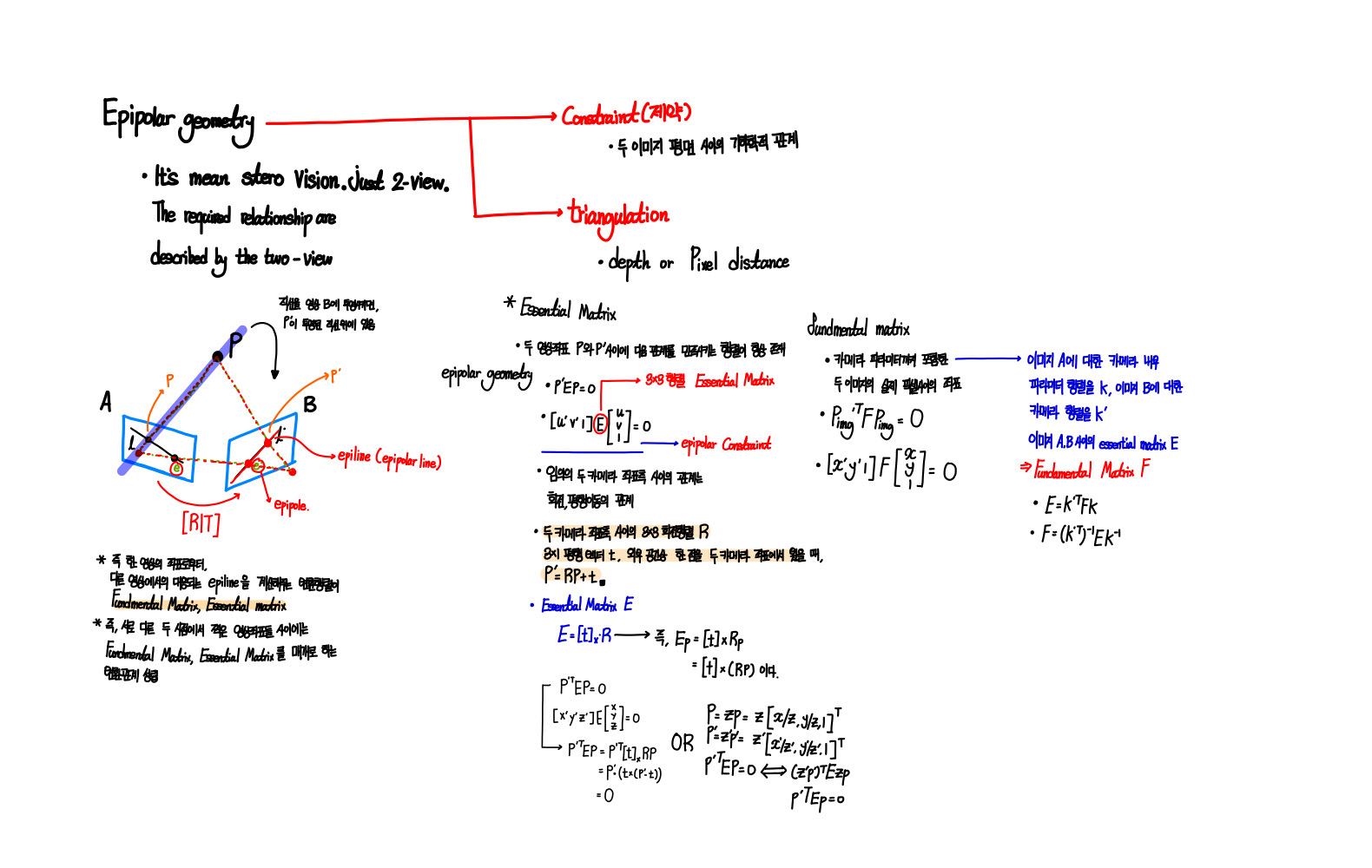
Reference
https://darkpgmr.tistory.com/83
'Study > Math' 카테고리의 다른 글
[Math] chapter 3.4 [Structure-from-Motion: Bundle Adjustment] (2) | 2023.04.08 |
---|---|
[Math] chapter 3.3 [Structure-from-Motion: Factorization] (0) | 2023.04.07 |
[Math] chapter 3.1 [Structure-from-Motion: Preliminaries] (0) | 2023.04.06 |
[Math] chapter 2.4 [Image Sensing Pipeline] (0) | 2023.04.06 |
[Math] chapter 2.3 [Image Formation] (0) | 2023.04.06 |