일 | 월 | 화 | 수 | 목 | 금 | 토 |
---|---|---|---|---|---|---|
1 | 2 | 3 | 4 | |||
5 | 6 | 7 | 8 | 9 | 10 | 11 |
12 | 13 | 14 | 15 | 16 | 17 | 18 |
19 | 20 | 21 | 22 | 23 | 24 | 25 |
26 | 27 | 28 | 29 | 30 | 31 |
- Vision
- nlp
- math
- Torch
- algorithm
- PRML
- classification
- pytorch
- clean code
- FGVC
- CV
- nerf
- FineGrained
- 딥러닝
- SSL
- computervision
- Front
- cs
- 머신러닝
- ML
- REACT
- 알고리즘
- Meta Learning
- Python
- web
- 3d
- GAN
- 자료구조
- dl
- Depth estimation
- Today
- Total
목록Depth estimation (4)
KalelPark's LAB
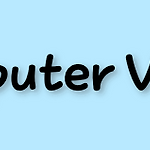
Depth Estimations Metrics Depth estimation에서 주로 사용하는 지표로는 5가지가 존재합니다. 1. Absolute Relative Error 2. Sqaure Relative Error 3. Root mean Sqaure Error 4. Log scale RMSE 5. Accuracy under a threshold 일반적으로, Absolute Relative Error, Sqaure Relative Error, Root mean Sqaure Error, Log scale RMSE 은 전통적으로 Regression 모델의 성능을 측정하기 위해 사용된 Metric이며, Accuracy under threshold는 Depth estimation 모델의 Accuracy를 측정..
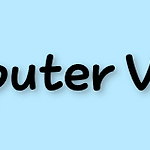
Abstract 다양한 scale로부터, depth에 대한 ground-truth를 얻는 것은 어렵습니다. 이러한 문제를 극복하기 위해, Self-Supervised Learning이 대안으로 떠오르고 있습니다. 본 논문에서는, 기존의 SSL 방법론에서 quantitatively와 qaulity를 개선한 방법을 제안합니다. 본 논문에서는, 단순한 Architecture를 보여줍니다. 주된 기여는. 1) robustly handle occulsions을 위한 mimum reprojection loss 2) visual artifact를 감소시키기 위한, full-resolution multi-scale sampling 방법 3) camera motion을 violate한 pixel을 무시하는 auto ma..
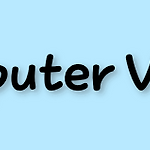
Abstract 본 논문에서는, monocular depth estimation과 관련한 새로운 접근법을 제시합니다. 이전의 방법과 유사하게, 우리의 방법론은 다른 CNN layer로부터, 파생된 multi scale information을 합치기 위해, continuous CRF를 사용합니다. 논문에서 제시하는 방법은, 기존의 방법과 다르게 다양한 feature들 사이의 저보들의 양을 자동으로 조절하는 structured attention model로부터, 많은 이득을 얻습니다. 본 논문에서 제시하는 방법은, CRF과 통합된 형태를 보이고, end-to-end로 학습하는 것이 가능합니다. Introduction 최근 CNN이 제안됨에 따라, 어떻게 estimate depth 와 semantic labe..
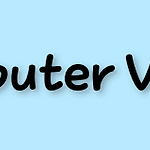
Abstract Learning based Method는 single Image로부터 depth를 추정하는데 상당히 좋은 결과를 보여주었지만, 기존의 접근법은 상당히 많은 데이터가 필요하다는 문제가 있습니다. 본 논문에서는, training동안에 데이터를 쉽게 얻을 수 있는 binocular stereo footage training을 사용함으로써, 기존의 depth data를 대체합니다. 우리는 새로운 training loss를 도입함으로써, 기존의 학습하는 동안 생성하는 disparity image의 quality를 향상시킵니다. 즉, 하나의 이미지를 좌우측에서의 비교하여, disparity를 파악함으로써, 성능을 개선하거나 기존의 방법론을 Robust하게 합니다. Introduction 기존의 De..