일 | 월 | 화 | 수 | 목 | 금 | 토 |
---|---|---|---|---|---|---|
1 | 2 | 3 | 4 | |||
5 | 6 | 7 | 8 | 9 | 10 | 11 |
12 | 13 | 14 | 15 | 16 | 17 | 18 |
19 | 20 | 21 | 22 | 23 | 24 | 25 |
26 | 27 | 28 | 29 | 30 | 31 |
- web
- dl
- Depth estimation
- PRML
- Python
- Torch
- classification
- cs
- ML
- FineGrained
- math
- FGVC
- 머신러닝
- algorithm
- Meta Learning
- GAN
- computervision
- 알고리즘
- Front
- pytorch
- CV
- nlp
- nerf
- clean code
- 자료구조
- 딥러닝
- SSL
- Vision
- REACT
- 3d
- Today
- Total
KalelPark's LAB
[Math] chapter 5.2 [Probabilistic Graphical Models : Markov Random Fields] 본문
[Math] chapter 5.2 [Probabilistic Graphical Models : Markov Random Fields]
kalelpark 2023. 4. 14. 11:31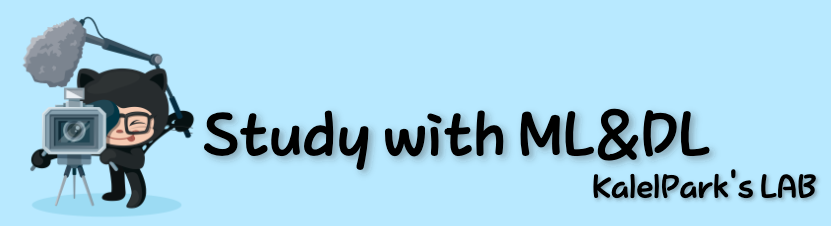
Markov Random Field, MRF
확률 그래프 모델로써 Maximum click에 대해서, Joint Probability로 표현한 것이다. 즉, 한 부분의 데이터를 알기 위해 전체의 데이터를 보고 판단하는 것이 아니라, 이웃하고 있는 데이터들과의 관계를 통해서 판단합니다.
[활용 분야]
- Imge Restoration (이미지 복원) - texture analysis (텍스쳐 분석)
- Image segmentation (이미지 분할) - Imge Labeling (이미지 레이블 정의)
- Edge detection (모서리 검출) - object recognition (물체 인식)
MRF를 알기 전에 2가지 그래프에 대해서 알고 넘어갈 필요가 있다.
Directed Graph vs Undirected Graph
Directed Graph
- edge를 통해서 노드들(Random Variables) 사이에 인과성이 주어짐.
- Bayesian Network에서 봤듯이 결합 확률 분포 P를 조건부 확률로 나타낼 수 있음.
Undirected Graph
- 노드들 사이에 대등한 상관관계가 주어짐.
- 조건부 확률을 쓸 수 없으므로, Potential function을 통해 분포를 나타냄
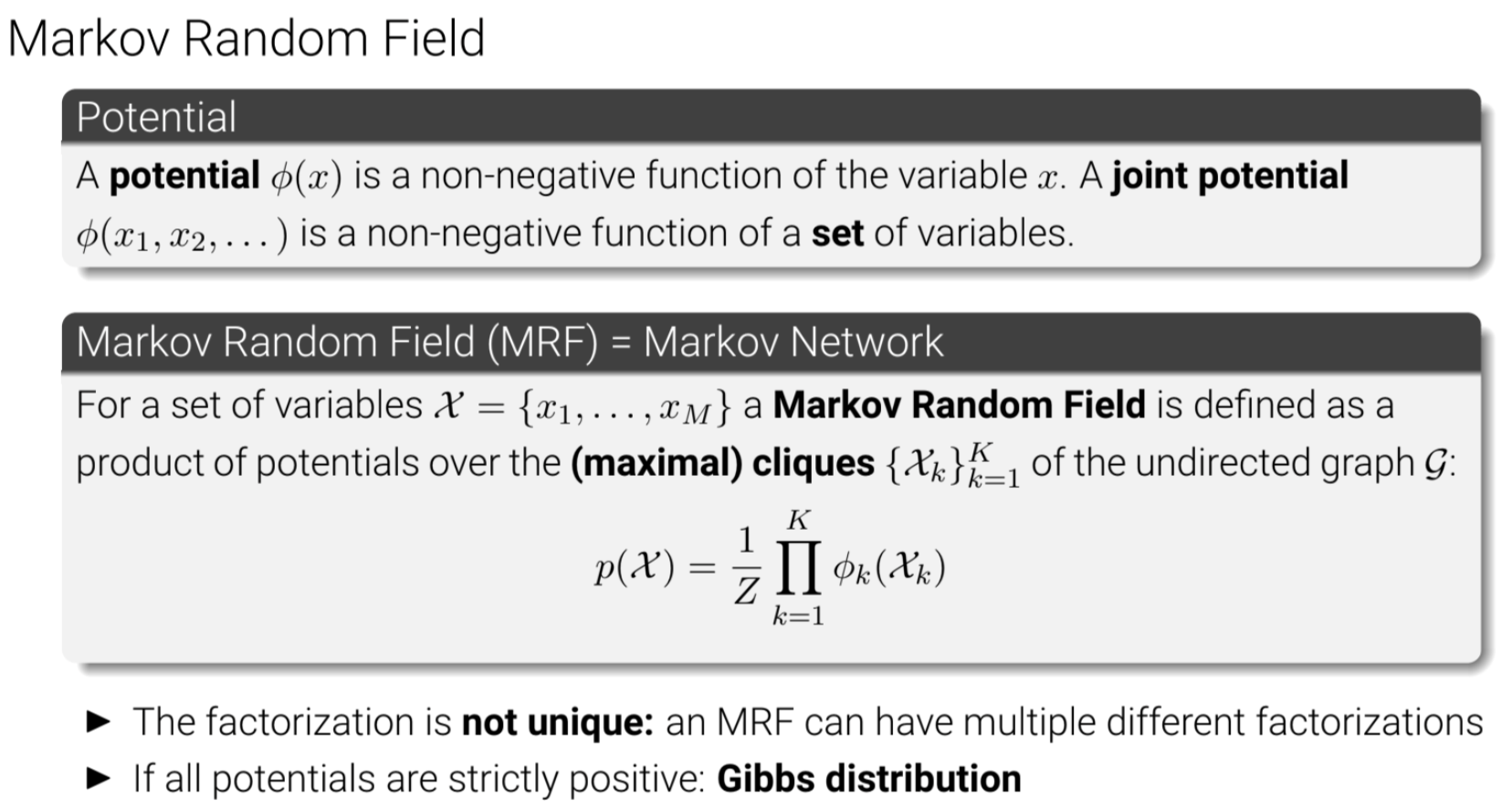
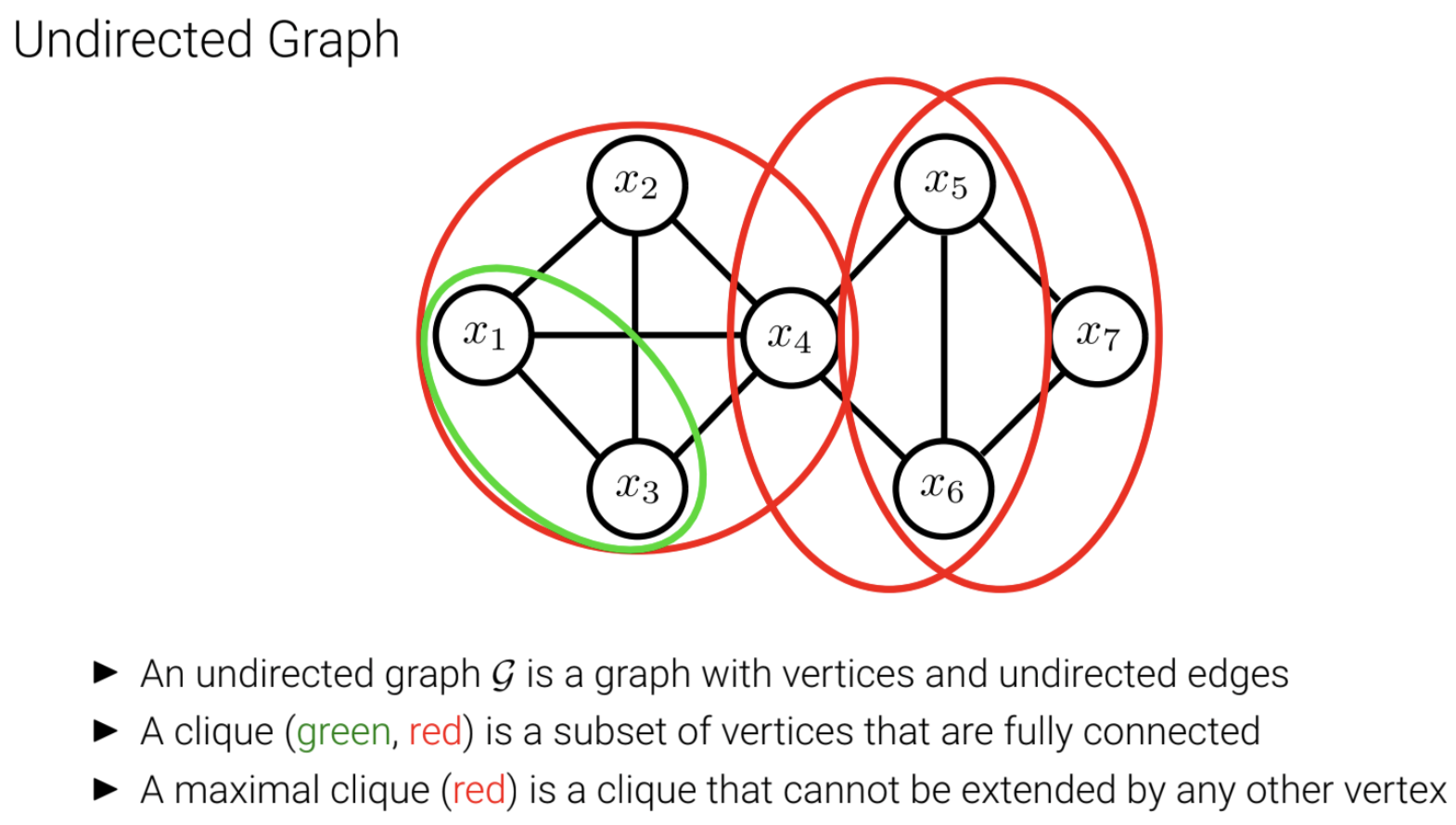
Properties of Markov Random Fields
노드들관의 관계를 파악하는 방법을 설명하고 있음.
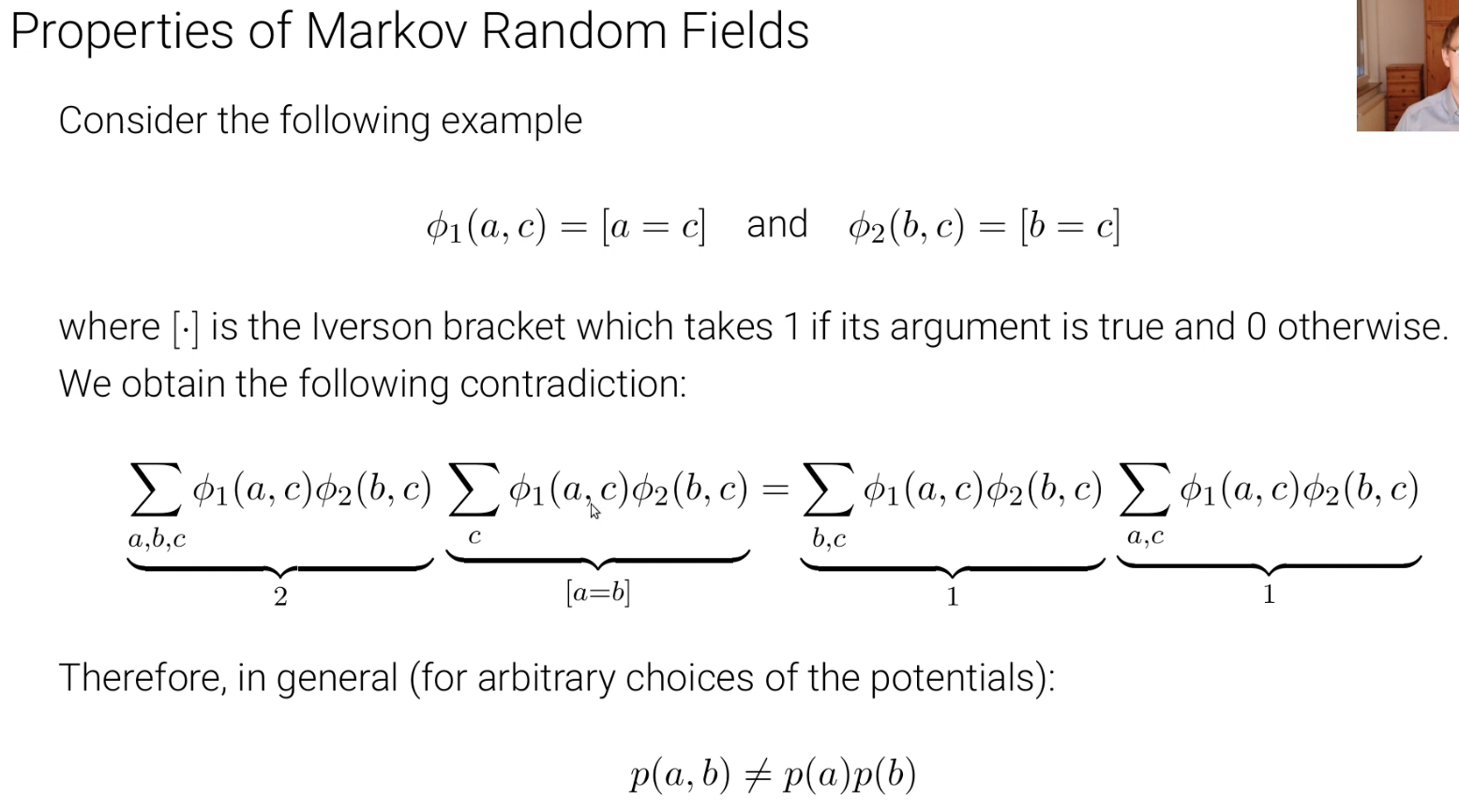
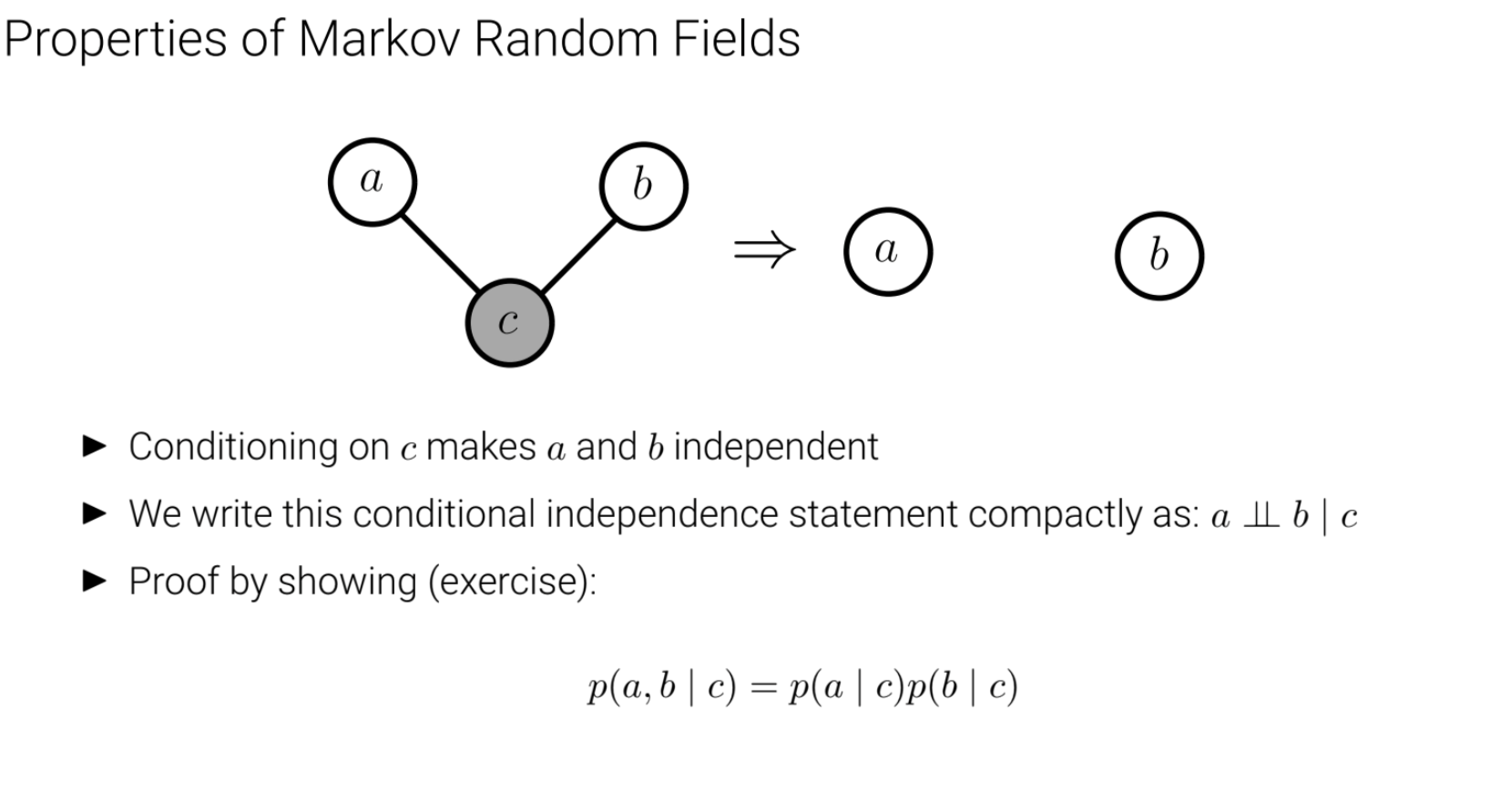
Global Markov Property과 Local Markov Property를 설명하고, Global Markov Property로부터, Local Markov Property를 제시함.
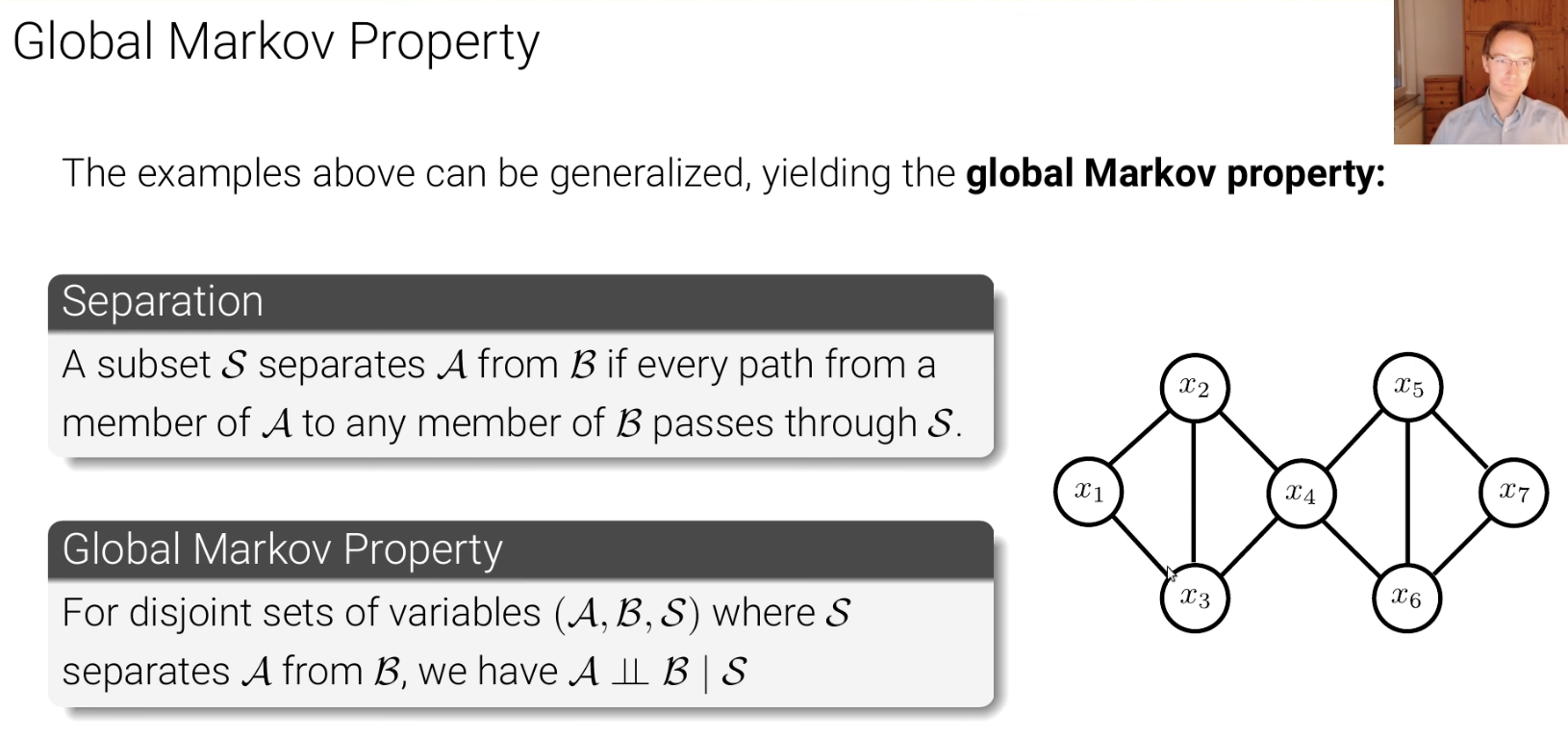

Filter View에서 어떻게 활용가능한지를 언급함.
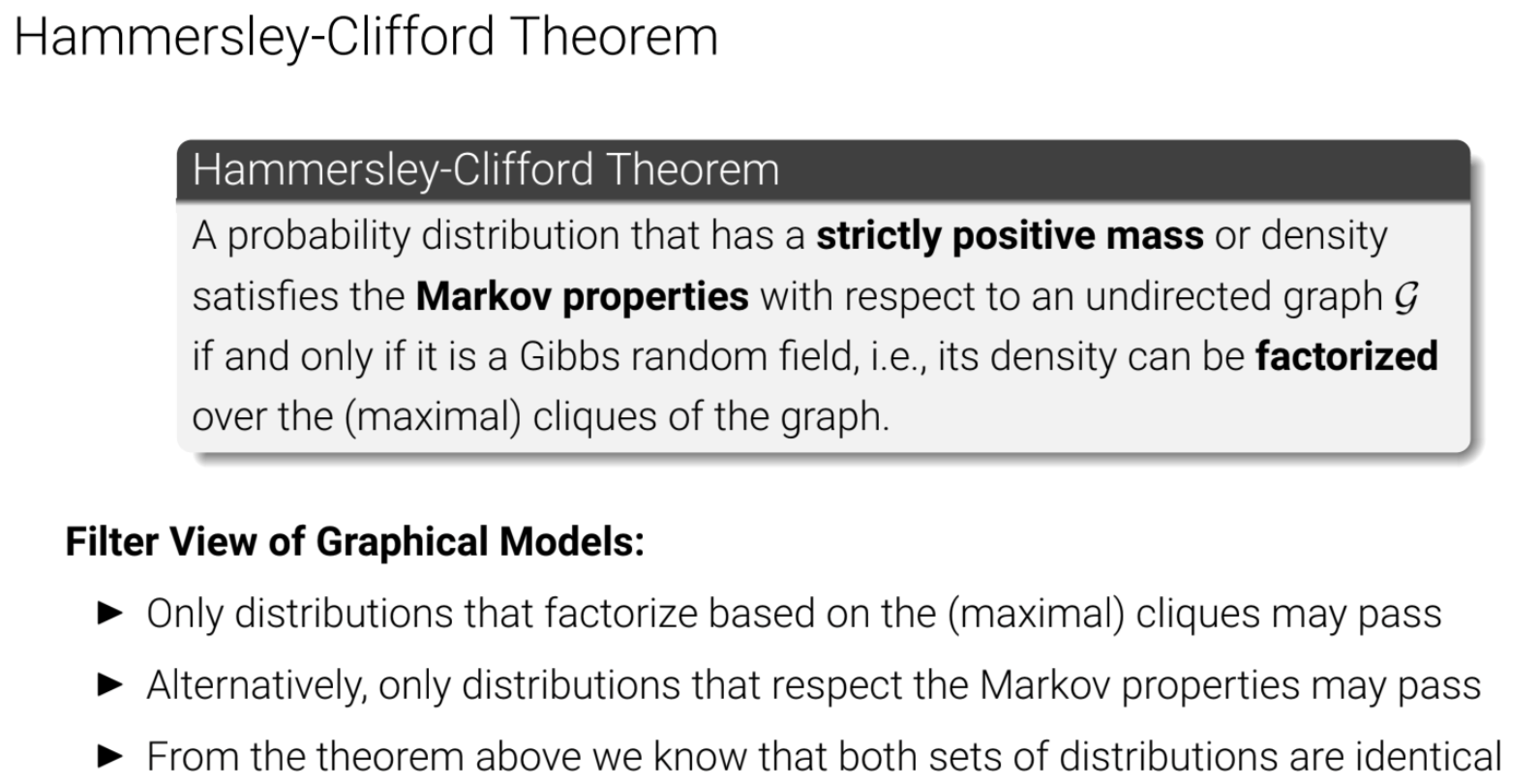
* 수학적인 부분은 추가적으로 포스팅 예정
Reference
https://benkyo-engineering.tistory.com/31
'Study > Math' 카테고리의 다른 글
[Math] Chi-Sqaured, T, F distribution (0) | 2023.04.16 |
---|---|
[Math] chapter 5.3 [Probabilistic Graphical Models : Factor Graphs] (0) | 2023.04.15 |
[Math] Monte Carlo & Markov Chain (0) | 2023.04.13 |
[Math] chapter 5.1 [Probabilistic Graphical Models: Structured Prediction] (0) | 2023.04.12 |
[Math] chapter 4.5 [Stereo Reconstruction: End-to-End Learning] (1) | 2023.04.11 |